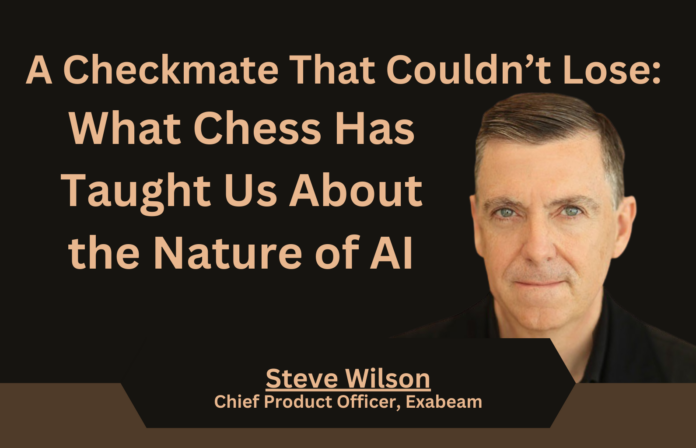
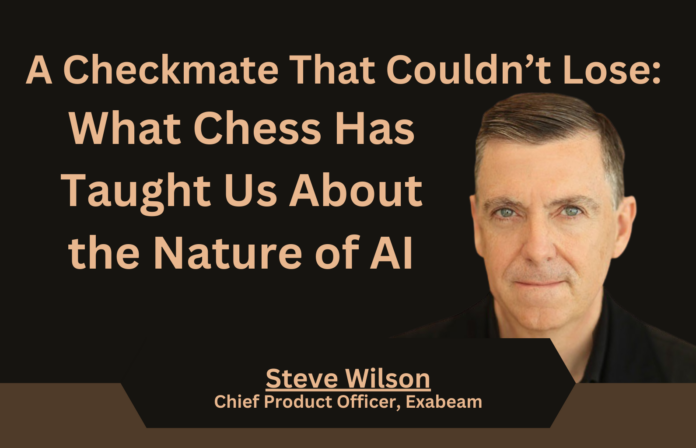
The best part about a competition is a worthy opponent, but what happens when the fellow contender is engineered to never lose?
The idea of artificial general intelligence (AGI) has emerged amid the artificial intelligence (AI) explosion. AGI is a theoretical pursuit to develop AI with human-like behavior such as self understanding, self-teaching, and a level of consciousness. AI technologies currently operate with a set of pre-determined parameters. For example, AI models can be trained to paraphrase content but often lack the reasoning abilities to generalize what they’ve learned.
While AGI may seem like a far-off ideal, it is closer than we think. The history of computerized chess algorithms can give us a glimpse into what might be right around the corner.
The Checkmates That Changed the World
Until the mid-20th century, chess was an area where human creativity and intuition reigned supreme over computers. In 1957, the first functional chess-playing program was developed at IBM, and by the 1980s, programs had achieved a level of play that rivaled even the greatest human chess minds. In 1989, IBM’s Deep Thought set the stage for computers to challenge some of the best human players when it defeated several grandmasters.
The 1990s saw two of the most famous competitions between humans and machines —with World Champion Garry Kasparov taking on IBM’s Deep Blue. In his initial match with Deep Blue in 1996, Kasparov emerged as the victor. But, in the 1997 rematch, the upgraded Deep Blue defeated Kasparov 3.5-2.5 — the first time a reigning champion lost to a computer. This event marked a turning point for AI and illustrated that machines could outperform humans in specific, deeply intellectual tasks.
Computer chess continued to evolve with the introduction of self-learning algorithms. Initially, chess engines relied heavily on human-crafted algorithms and databases of previous games. With the introduction of the self-learning algorithm AlphaZero, which used reinforcement learning to teach itself, we began to see a level of superhuman play. By playing millions of games against itself, learning and improving with each iteration, AlphaZero was able to surpass the capabilities of the world’s best engines like Stockfish in just a few hours. In a 100-game match against Stockfish, the best human-developed chess engine, AlphaZero went undefeated- winning 28 games and drawing 72.
Today, AlphaZero boasts standard Elo ratings of over 3500, while the best human players are only around 2850. The odds of a human champion defeating a top engine? Less than 1%. In fact, experts widely believe that no human will ever again beat an elite computer chess algorithm.
Learning to Expect Sudden Jumps in GenAI’ s Capabilities
Chess’ evolution offers valuable insights into the development of other AI technologies, particularly Generative AI (GenAI). Both fields have shifted from relying on human-crafted strategies to adopting self-learning systems.
Modern Large Language Models (LLMs), like GPT-4, can process vast amounts of data through unsupervised learning and perform a wide range of tasks autonomously. This suggests we are on the cusp of witnessing exponential growth in AGI. The progression we’ve seen—where slow, incremental advances suddenly give way to explosive improvements—serves as a clear indicator of AGI’s potential. With AGI, this may not only result in outperforming humans in specific tasks but rapidly evolving to handle a broader range of cognitive functions independently.
The technical drivers behind these leaps are already emerging. LLMs like GPT-4 have shown an ability to scale unsupervised learning, enhancing performance across multiple domains with minimal human input. The architecture’s ability to process and generate massive amounts of data in parallel accelerates the learning cycle. As these systems are provided with more computational power and data, the likelihood of rapid and dramatic improvements becomes even more probable.
This is not a gradual evolution but an exponential one. Once a general AI system reaches a critical threshold in its learning capabilities, it could swiftly surpass human intelligence across various fields. Preparing for this rapid inflection point is not only a technical challenge but also a strategic imperative for organizations seeking to leverage AI responsibly. That’s why establishing robust ethical frameworks and implementing technical safeguards now is essential.
Unsupervised AI Learning in the Real World
While AI-powered chess may be all fun and games (pun intended), the implications in the real world of autonomous learning, a giant step toward AGI, are far from benign. Here are a few examples:
1.In 2016, Microsoft’s AI Chatbot for Twitter, Tay, turned quickly offensive when exposed to unfiltered data. Soon after it launched, people started communicating with the bot using misogynistic and racist content. Tay learned from these conversations and began repeating similar-sounding statements back to users.
2.A few months after ChatGPT was launched, adversaries began claiming that they had used the technology to create malware, phishing emails, or powerful social engineering campaigns.
3.When the U.S. military began integrating AI into wargames, they were surprised to see that the preferred outcomes from OpenAI’s technology were extremely violent. In multiple replays of a simulation, the AI chose to launch nuclear attacks.
We’ve opened Pandora’s box, and can’t shut it again — so what do we do?
Reconciling the Benefits of AI With Its Potential Risks
At every step of technological advancements, there has been fearmongering and concern. While some trepidation is valid, we can’t return to a world where AI does not exist — nor should we want to. To reap the benefits of AI (and even AGI), we must address ethical concerns and build robust security frameworks.
Here’s my call to action for organizations:
1. Experiment with Gen AI now. The fear is largely in the unknown. Get to know how AI can benefit your organization and begin to get comfortable with the technology.
2. Learn about the risks. It’s no secret that AI comes with several security risks. Security teams should dedicate time to learning about the latest threats. The OWASP Top 10 for Large Language Models is a great place to start.
3. Prepare a policy on Gen AI. Have representatives from each department of your organization come together to determine how you use Gen AI. Decide which apps are okay versus not okay. Then, write it down and share it with the whole company so everyone is on the same page.
Chess showed us what AGI might look like in the future. By acknowledging the dangers of AI and taking the right steps to protect ourselves, we can ride the tidal wave of innovation rather than be caught in the undertow. After all, a little challenge from a worthy opponent presents us with an opportunity to learn and improve.